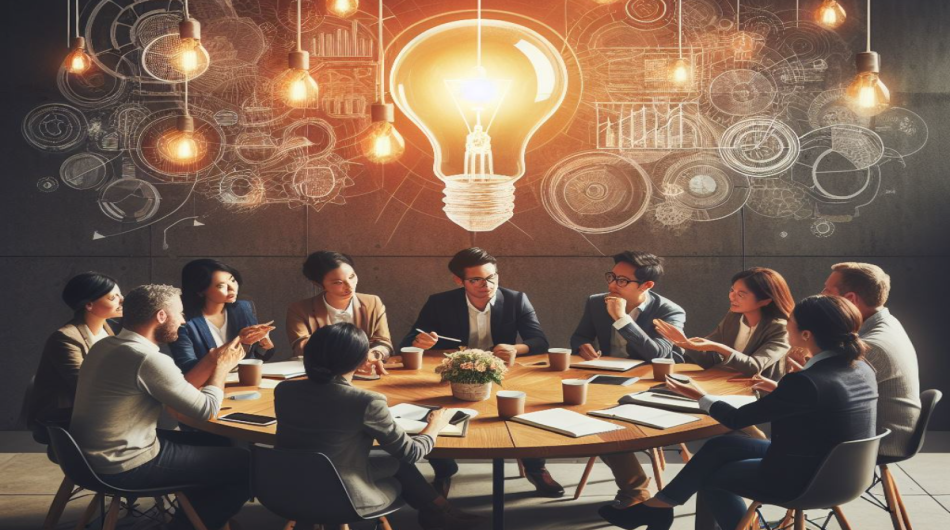
Aggregated Intelligence (AI) is an emerging concept that combines the power of artificial intelligence, machine learning, and data analytics to harness the collective knowledge, insights, and problem-solving abilities of diverse groups of people and machines. By integrating human intelligence with computational tools and platforms, Aggregated Intelligence aims to tackle complex challenges, make better decisions, and drive innovation across various domains, from business and healthcare to science and governance. This article provides a comprehensive overview of Aggregated Intelligence, its key principles, technologies, applications, challenges, and future prospects.
Defining Aggregated Intelligence
Aggregated Intelligence is a term that encompasses a range of approaches and methodologies for combining human and machine intelligence to achieve outcomes that exceed the capabilities of either alone. At its core, Aggregated Intelligence is based on the idea that the collective intelligence of a group can be harnessed and amplified through the use of digital technologies, such as crowdsourcing platforms, collaborative tools, and data analytics.
The concept of Aggregated Intelligence draws from various fields, including:
-
Collective Intelligence: The study of how groups of individuals can act intelligently and make decisions that are better than those of any single member.
-
Crowdsourcing: The practice of obtaining information, ideas, or services from a large group of people, typically via the internet.
-
Human Computation: The use of human intelligence to perform tasks that are difficult for computers, such as image recognition or sentiment analysis.
-
Machine Learning: The development of algorithms and statistical models that enable computers to learn and improve their performance on a specific task without being explicitly programmed.
By combining these approaches, Aggregated Intelligence seeks to create synergies between human and machine capabilities, leveraging the strengths of each to solve problems, generate insights, and drive innovation.
Key Principles of Aggregated Intelligence
Aggregated Intelligence is based on several key principles that guide its design and implementation:
-
Diversity: Aggregated Intelligence relies on the diversity of perspectives, knowledge, and skills within a group to generate novel ideas and solutions. By bringing together individuals with different backgrounds, expertise, and experiences, Aggregated Intelligence can overcome the limitations of individual biases and blind spots.
-
Collaboration: Effective Aggregated Intelligence requires collaboration and communication among participants, as well as between humans and machines. By creating an environment that fosters open sharing of information, ideas, and feedback, Aggregated Intelligence can facilitate collective learning and problem-solving.
-
Incentives: To motivate participation and engagement, Aggregated Intelligence systems often incorporate incentives, such as rewards, recognition, or gamification elements. These incentives can help to align the interests of participants with the goals of the system and encourage high-quality contributions.
-
Aggregation: A central component of Aggregated Intelligence is the aggregation of individual contributions into a collective output. This can involve various methods, such as voting, ranking, filtering, or machine learning algorithms, depending on the nature of the task and the desired outcome.
-
Iteration: Aggregated Intelligence is an iterative process that involves continuous feedback, learning, and refinement. By incorporating mechanisms for testing, evaluation, and adaptation, Aggregated Intelligence systems can evolve and improve over time, based on the input and insights of participants.
Benefits and Challenges of Aggregated Intelligence
Aggregated Intelligence offers several potential benefits for organizations and society:
-
Improved decision-making: By leveraging the collective knowledge and insights of diverse groups, Aggregated Intelligence can help organizations make more informed and robust decisions, reducing the risk of individual biases or errors.
-
Increased innovation: Aggregated Intelligence can facilitate the generation of novel ideas and solutions by bringing together different perspectives and expertise, leading to breakthrough innovations and creative problem-solving.
-
Enhanced efficiency: By automating certain tasks and leveraging the power of machine learning, Aggregated Intelligence can help organizations to streamline processes, reduce costs, and improve overall efficiency.
-
Greater inclusivity: Aggregated Intelligence can enable the participation of a wider range of stakeholders in decision-making and problem-solving, including customers, employees, and citizens, leading to more inclusive and democratic outcomes.
However, Aggregated Intelligence also faces several challenges and limitations:
-
Quality control: Ensuring the quality and reliability of contributions from diverse participants can be challenging, particularly in large-scale systems where manual review is not feasible.
-
Bias and fairness: Aggregated Intelligence systems can perpetuate or amplify existing biases and inequalities if not designed and implemented with attention to diversity, inclusion, and fairness.
-
Privacy and security: The collection, sharing, and analysis of large amounts of data in Aggregated Intelligence systems raise concerns about privacy, data protection, and cybersecurity.
-
Coordination and governance: Managing the interactions and contributions of large numbers of participants, as well as ensuring alignment with organizational goals and values, requires effective coordination and governance structures.
Addressing these challenges requires a multidisciplinary approach that combines technical, social, and organizational strategies, as discussed in later sections of this article.
Technologies and Platforms for Aggregated Intelligence
The implementation of Aggregated Intelligence relies on a range of technologies and platforms that enable the collection, processing, and analysis of data from diverse sources, as well as the coordination and collaboration among participants. Some of the key technologies and platforms used in Aggregated Intelligence include:
Crowdsourcing Platforms
Crowdsourcing platforms are online environments that enable organizations to engage large numbers of people in various tasks, such as idea generation, problem-solving, or data collection. These platforms typically provide tools for posting challenges, receiving submissions, and managing interactions among participants. Examples of crowdsourcing platforms used in Aggregated Intelligence include:
-
Amazon Mechanical Turk: A marketplace for businesses to outsource tasks that require human intelligence, such as image classification, data verification, or content moderation.
-
InnoCentive: A platform that connects organizations with a global network of problem-solvers to tackle complex challenges in fields such as engineering, science, and business.
-
Kaggle: A community of data scientists and machine learning practitioners that compete to solve real-world problems and build predictive models using shared datasets.
Collaboration and Communication Tools
Effective Aggregated Intelligence requires tools that facilitate collaboration and communication among participants, enabling them to share information, ideas, and feedback in real-time. These tools can range from basic messaging and file-sharing applications to more sophisticated platforms that support structured workflows and decision-making processes. Examples of collaboration and communication tools used in Aggregated Intelligence include:
-
Slack: A cloud-based messaging platform that enables teams to communicate and collaborate in real-time, with features such as channels, direct messages, and file sharing.
-
Microsoft Teams: A unified communication and collaboration platform that combines chat, video meetings, file storage, and application integration.
-
Google Workspace: A suite of cloud-based productivity and collaboration tools, including Gmail, Google Drive, Google Docs, and Google Meet.
Data Analytics and Machine Learning Platforms
Aggregated Intelligence relies heavily on data analytics and machine learning to process and derive insights from large amounts of structured and unstructured data. These platforms provide tools for data integration, cleaning, exploration, and modeling, as well as visualization and reporting capabilities. Examples of data analytics and machine learning platforms used in Aggregated Intelligence include:
-
Tableau: A data visualization and business intelligence platform that enables users to connect, analyze, and share data from various sources.
-
IBM Watson: A suite of AI services and applications that enable organizations to build and deploy intelligent solutions, such as natural language processing, computer vision, and predictive analytics.
-
TensorFlow: An open-source machine learning framework developed by Google that allows users to build and train neural networks for various tasks, such as image recognition, language translation, and time series forecasting.
Blockchain and Distributed Ledger Technologies
Blockchain and distributed ledger technologies (DLTs) are emerging as potential enablers of Aggregated Intelligence, particularly in contexts where trust, transparency, and security are critical. These technologies provide a decentralized and immutable record of transactions and interactions among participants, enabling secure and auditable data sharing and collaboration. Examples of blockchain and DLT platforms used in Aggregated Intelligence include:
-
Ethereum: A decentralized, open-source blockchain platform that enables the development of smart contracts and distributed applications (dApps).
-
Hyperledger Fabric: A permissioned blockchain framework hosted by the Linux Foundation that enables the development of enterprise-grade blockchain solutions.
-
IOTA: A distributed ledger platform designed for the Internet of Things (IoT) that enables secure and scalable data exchange and transaction settlement.
The integration and orchestration of these various technologies and platforms are crucial for the effective implementation of Aggregated Intelligence systems, as discussed in the next section.
Applications and Use Cases of Aggregated Intelligence
Aggregated Intelligence has a wide range of potential applications across various domains, from business and finance to healthcare and science. Some of the most prominent use cases of Aggregated Intelligence include:
Business and Finance
In the business and finance domain, Aggregated Intelligence can be used to improve decision-making, risk management, and customer engagement. For example:
-
Predictive analytics: Aggregated Intelligence can help organizations to predict market trends, customer behavior, and financial risks by combining data from various sources, such as social media, transaction records, and sensor networks.
-
Customer insights: By analyzing customer feedback, reviews, and interactions across multiple channels, Aggregated Intelligence can provide a more comprehensive understanding of customer needs, preferences, and sentiments.
-
Fraud detection: Aggregated Intelligence can help financial institutions to detect and prevent fraudulent activities by leveraging machine learning algorithms and collective intelligence from experts and customers.
Healthcare and Life Sciences
In the healthcare and life sciences domain, Aggregated Intelligence can be used to improve patient outcomes, accelerate drug discovery, and support clinical decision-making. For example:
-
Precision medicine: By combining data from electronic health records, genomic databases, and wearable devices, Aggregated Intelligence can enable personalized diagnosis, treatment, and prevention strategies.
-
Drug discovery: Aggregated Intelligence can accelerate the identification of new drug targets and candidates by leveraging the collective knowledge of researchers, clinicians, and patients, as well as machine learning algorithms.
-
Clinical decision support: By integrating data from various sources, such as clinical guidelines, research literature, and patient histories, Aggregated Intelligence can provide evidence-based recommendations to support clinical decision-making.
Science and Research
In the science and research domain, Aggregated Intelligence can be used to accelerate discovery, enable large-scale collaboration, and facilitate knowledge sharing. For example:
-
Citizen science: Aggregated Intelligence can enable the participation of non-experts in scientific research by providing platforms for data collection, analysis, and interpretation, such as online forums, mobile apps, and gaming environments.
-
Collaborative research: By leveraging collaboration tools and data-sharing platforms, Aggregated Intelligence can facilitate multi-disciplinary and multi-institutional research projects, enabling researchers to share data, methods, and insights across geographical and organizational boundaries.
-
Knowledge discovery: Aggregated Intelligence can help to uncover novel insights and hypotheses by combining data from various sources, such as scientific publications, experimental datasets, and simulation models, and applying machine learning and natural language processing techniques.
Government and Public Policy
In the government and public policy domain, Aggregated Intelligence can be used to improve public services, enhance citizen engagement, and support evidence-based policymaking. For example:
-
Smart cities: By leveraging data from various sources, such as sensor networks, social media, and government databases, Aggregated Intelligence can enable the development of data-driven solutions for urban challenges, such as traffic congestion, energy efficiency, and public safety.
-
Participatory governance: Aggregated Intelligence can enable the participation of citizens in decision-making processes by providing platforms for online deliberation, voting, and feedback, such as e-petitions, participatory budgeting, and policy consultations.
-
Policy analysis: By combining data from various sources, such as economic indicators, social surveys, and research studies, Aggregated Intelligence can support the development and evaluation of public policies, enabling policymakers to make more informed and evidence-based decisions.
These are just a few examples of the potential applications of Aggregated Intelligence, and the list is likely to grow as the field continues to evolve and mature.
Challenges and Future Directions
Despite the significant potential of Aggregated Intelligence, there are several challenges and open questions that need to be addressed to realize its full benefits. Some of the key challenges and future directions for Aggregated Intelligence include:
Technical Challenges
-
Scalability and performance: As the volume and complexity of data and interactions in Aggregated Intelligence systems increase, ensuring their scalability and performance becomes a critical challenge. This requires the development of efficient algorithms, distributed architectures, and cloud-based solutions that can handle large-scale data processing and real-time collaboration.
-
Interoperability and standards: To enable seamless integration and collaboration among different Aggregated Intelligence systems and platforms, there is a need for common standards and protocols for data exchange, API design, and security. This requires the development of industry-wide consortia and open-source initiatives that can promote interoperability and foster innovation.
-
Explainability and transparency: As Aggregated Intelligence systems become more complex and autonomous, ensuring their explainability and transparency becomes crucial for building trust and accountability. This requires the development of methods and tools for interpreting and communicating the reasoning behind the decisions and recommendations made by these systems.
Social and Ethical Challenges
-
Bias and fairness: Aggregated Intelligence systems can perpetuate or amplify existing biases and inequalities if not designed and implemented with attention to diversity, inclusion, and fairness. This requires the development of methods and metrics for detecting and mitigating bias, as well as the promotion of diversity and inclusivity in the design and governance of these systems.
-
Privacy and security: The collection, sharing, and analysis of large amounts of data in Aggregated Intelligence systems raise concerns about privacy, data protection, and cybersecurity. This requires the development of secure and privacy-preserving technologies, such as differential privacy, homomorphic encryption, and secure multi-party computation, as well as the establishment of legal and ethical frameworks for data governance.
-
Accountability and responsibility: As Aggregated Intelligence systems become more autonomous and influential, questions arise about who is responsible and accountable for their actions and outcomes. This requires the development of clear and transparent mechanisms for assigning responsibility and liability, as well as the promotion of ethical and responsible AI practices.
Future Research Directions
-
Human-AI collaboration: A key direction for future research in Aggregated Intelligence is to develop more effective and intuitive ways for humans and AI systems to collaborate and complement each other's strengths. This includes the design of user interfaces, interaction protocols, and feedback mechanisms that can facilitate seamless and productive collaboration between humans and machines.
-
Decentralized and self-organizing systems: Another promising direction for Aggregated Intelligence is the development of decentralized and self-organizing systems that can operate without central control or coordination. This includes the use of blockchain and smart contract technologies to enable secure and transparent collaboration among participants, as well as the design of emergent and adaptive algorithms that can learn and evolve based on the collective behavior of the system.
-
Hybrid intelligence: A further direction for Aggregated Intelligence is the integration of different types of intelligence, such as symbolic, sub-symbolic, and embodied intelligence, to create more flexible and robust systems that can handle a wider range of tasks and environments. This includes the development of hybrid architectures that combine rule-based, statistical, and neural approaches, as well as the design of multi-modal interfaces that can process and generate information in various forms, such as text, speech, and images.
Addressing these challenges and exploring these future directions requires a multidisciplinary approach that brings together researchers, practitioners, and stakeholders from various fields, such as computer science, social science, psychology, and ethics. It also requires the development of new methodologies, tools, and frameworks that can support the design, implementation, and evaluation of Aggregated Intelligence systems in a responsible and trustworthy manner.
Conclusion
Aggregated Intelligence is an exciting and rapidly evolving field that has the potential to transform the way we solve complex problems, make decisions, and create value in various domains. By combining the power of human and machine intelligence, Aggregated Intelligence can enable us to tackle challenges that are beyond the capabilities of either alone, and to generate insights and solutions that are more diverse, creative, and robust.
However, realizing the full potential of Aggregated Intelligence also requires addressing significant technical, social, and ethical challenges, such as ensuring scalability, interoperability, fairness, privacy, and accountability. This requires a collaborative and interdisciplinary effort that engages researchers, practitioners, policymakers, and citizens in the design, development, and governance of Aggregated Intelligence systems.
As we look to the future, we can expect Aggregated Intelligence to play an increasingly important role in shaping our world, from enabling scientific breakthroughs and technological innovations to supporting social and environmental sustainability. By harnessing the collective intelligence of humans and machines, we can create a more informed, inclusive, and resilient society that can adapt and thrive in the face of complex and ever-changing challenges.
To realize this vision, we need to continue to invest in research, education, and innovation in Aggregated Intelligence, and to foster a culture of collaboration, transparency, and responsibility. We also need to engage in broader public dialogues and deliberations about the ethical and societal implications of Aggregated Intelligence, and to develop governance frameworks that can ensure its beneficial and responsible development and use.
In conclusion, Aggregated Intelligence represents a powerful and promising approach to harnessing the collective intelligence of humans and machines for the betterment of society. By combining the best of both worlds, we can create a future that is more intelligent, inclusive, and sustainable, and that empowers us to solve the most pressing challenges of our time.